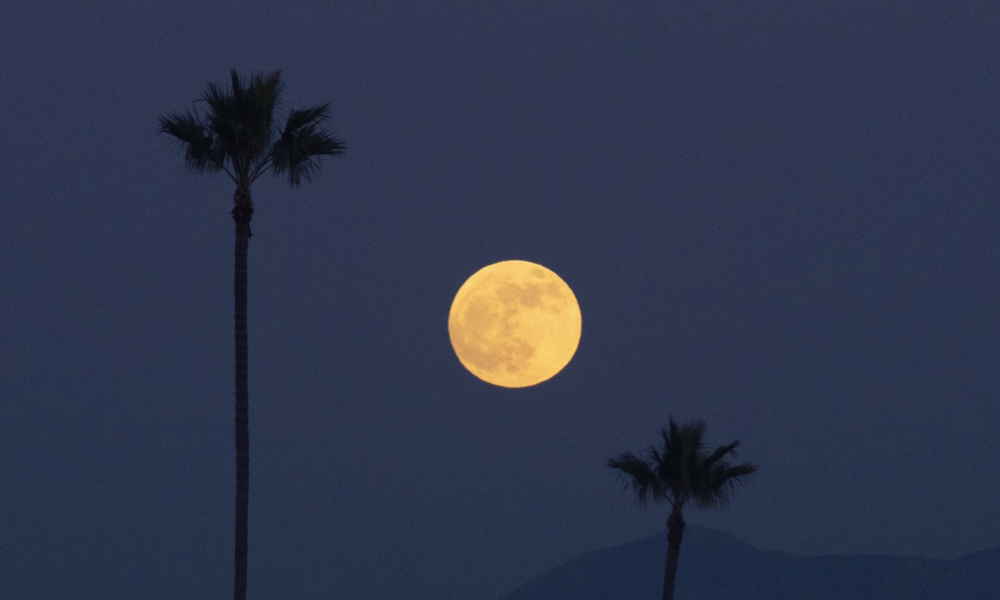
ESA Open Invitation to Tender AO10151
Open Date: 24/01/2020
Closing Date: 20/03/2020 13:00:00
Status: ISSUED
Reference Nr.: 19.154.24
Prog. Ref.: NAVISP Phase 1 E1
Budget Ref.: E/0365-10 – NAVISP Phase 1 E1
Special Prov.: BE+DK+DE+CH+FR+GB+AT+NL+NO+FI+CZ+RO+GR+IT+SE+HU
Tender Type: C
Price Range: 200-500 KEURO
Products: Ground Segment / Mission Operations / Mission Control / Satellite and Ground Segment Simulators (e.g. simsat, eurosim, etc.)
Technology Domains: Flight Dynamics and GNSS / GNSS High-Precision Data Processing / GNSS and Geodetic Data Processing
Establishment: ESTEC
Directorate: Directorate of Navigation
Department: Strategy and Programme Department
Division: NAVISP Programme Office
Contract Officer: Karger-Kocsis, Anna
Industrial Policy Measure: N/A – Not apply
Last Update Date: 24/01/2020
Update Reason: Tender issue
This activity shall aim at overcoming some of the limitations of current macro model based engineering tools by training a machine learning (ML) algorithm to predict GNSS system performances. In general, the GNSS navigation chain is composed of a network of GNSS sensors aiming at collecting some data from a space segment (core-constellation of satellites), and a Kernel implementing the navigation algorithms processing this data to produce a navigation message. This Kernel represents the brain of the navigation chain and the objective of the activity is to develop an ML algorithm predicting its behaviour. To carry out its task, the ML algorithm will have to read the values of some metrics reflecting the quality of the data produced by the GNSS sensors. Trained on years of real data, the created tool shall be an intermediary product between the two existing simulation options. With the user-friendly operability and low computational cost of current macro model tools, it shall offer more accurate results on a wider range of different scenarios than a macro model which only accurately represents scenarios close to the tuned scenario. Aside from absolute system performance,another important aspect in design decisions is the system’s sensitivity to single factors like GNSS sensors positions. An advantageof using ML are the data analysis capabilities that come with it. Within the scope of this study, these techniques shall be used tostudy the sensitivity of the algorithm output, the navigation message, to single input parameters. As ML has so far not been used to simulate GNSS system performances, this study aims as well at breaking the ground by demonstrating the feasibility of the technology, enabling many more possible applications within the PNT sector and beyond.
If you wish to access the documents related to the Invitation to Tender, you have to log in to the ESA Portal.