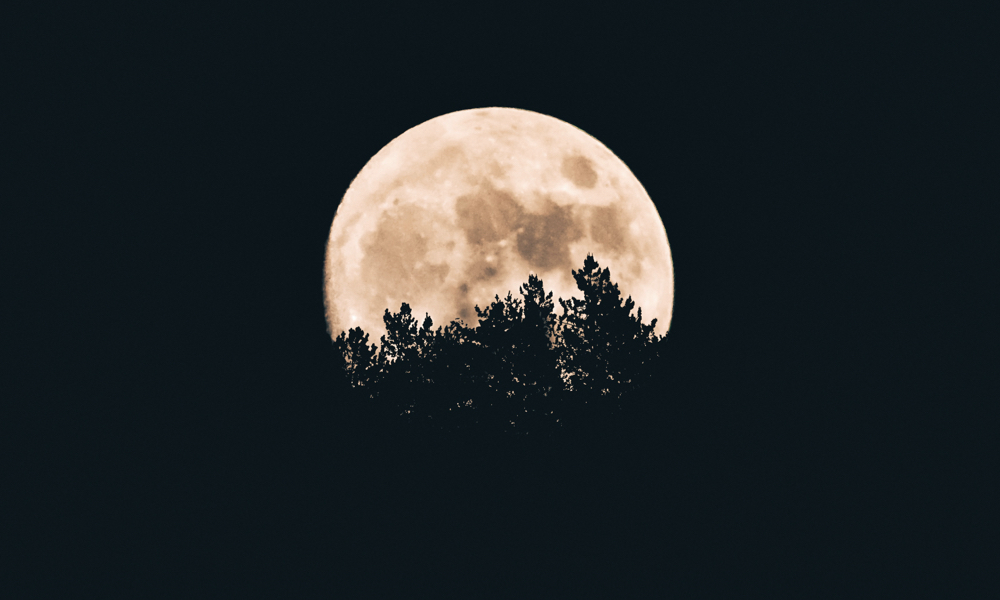
ESA Open Invitation to Tender: 1-10839
Open Date: 06/09/2021 17:15 CEST
Closing Date: 01/11/2021 13:00 CEST
Recent developments in AI and Machine learning research have proven that many different models of inference system have potential use in next generation space missions for on-board data analysis; autonomous decision making; sensor diagnostic and prognostic; etc. These methods are highly flexible computational tools supported by energy efficient hardware accelerators. There is still an unsolved problem to explain the relationships between their inputs and their final outputs, especially on AI systems based on deep learning techniques. The inability to backtrack the inference process in a meaningful way hinders the adoption of AI models for safety-critical systems which require the inference process to be manifest and non-ambiguous, possibly supported by well defined mathematical models. Machine learning systems are not robust by default. Even systems that outperform humans in a particular domain can fail at solving simple problems if subtle differences are introduced. For dependable systems we need a solution that evaluates the AI/ML network as a black box algorithm already trained to perform a specific task, and evaluates the ‘corner cases’ in (for example) image analysis. The idea is to develop a modular framework that can be adopted for multiple analyses. We need to supplement next AI inference systems with a set of operational conditions and ranges of tolerance for user-defined input distortions that may serve as a first step in defining the robustness of the system to errors and faults, possibly bridging the gap between safety-critical systems and AI systems towards the adoption of AI engines in future space applications. The core this activity is on the set-up of a full end-to-end tool-chain and neuralnetwork inference framework that can be trained on-ground (e.g. cloud based) and then be deployed and executed on a typical flight system, with particular care on fault tolerant techniques and verification and validation problems, impacting use of AI/ML in all relevant space missions.Tasks: Definition of ML use cases in mission critical applications (example rover guidance, star tracking, FDIR management, autonomous collisionavoidance manoeuvres); Definition of target hardware (Accelerators, FPGA, processors) and of suitable FT techniques to be adopted; Development of an Engineering Model, using representative space gradedHW. Verification and validation of the developed EM.
Directorate: Directorate of Tech, Eng. Quality
Estabilishment: ESTEC
ECOS Required: No
Classified: No
Price Range: > 500 KEURO
Authorised Contact Person: Anze Singer
Initiating Service: TEC-EDD
IP Measure: N/A
Prog. Reference: E/0904-611 – GSTP Element 1 Dev
Tender Type: Open Competition
Open To Tenderers From: DE
Technology Keywords: 1-A-II-Hardware Technologies for Payload Data Processing / 1-B-II-Onboard Computers / 9-A-Advanced System Concepts / 9-C-III-Human–Computer Interfaces and Technologies / 13-A-I-Planetary Exploration
Products Keywords: 2-E-3-a-File management systems / 2-F-1.1-a-General Purpose Microprocessors (ERC32, Leon 2) / 2-F-1.1-c-Microcontrollers / 2-F-1.1-d-Dedicated Signal Processing Processors (e.g. FFT, Compression) / 2-F-1.1-i-TM/TC (Formater, encryption)
If you wish to access the documents related to the Invitation to Tender, you have to log in to the ESA Portal.