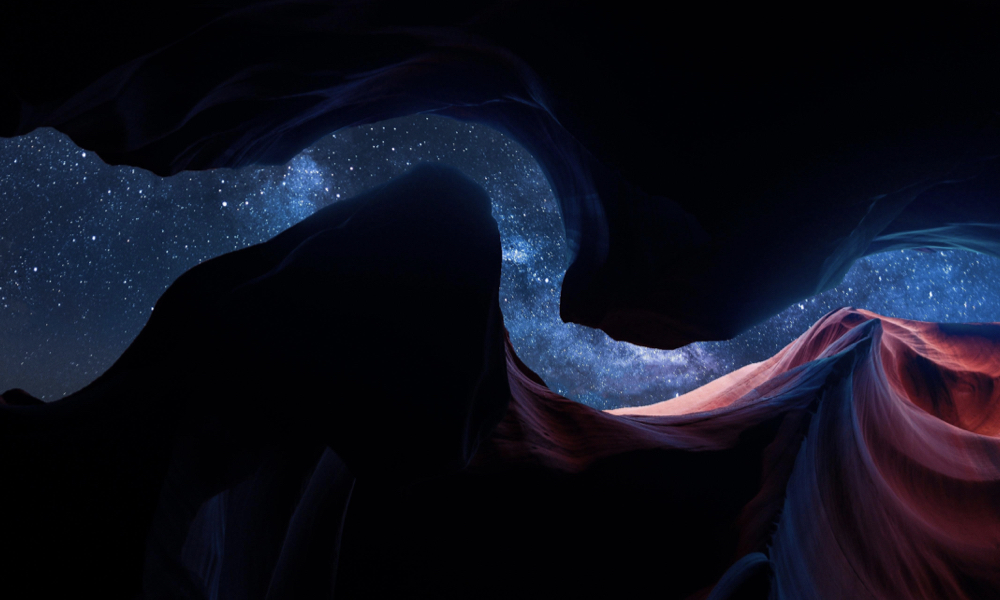
ESA Open Invitation to Tender: 1-10711
Open Date: 20/04/2021 13:24 GMT +3
Closing Date: 01/06/2021 13:00 GMT +3
Spacecraft’s produce an ever-increasing amount of data for increased scientific output and better monitoring and operations capabilities. Due to limited on-boardcomputational power, most of the processing and analysis is performed on-ground, requiring all raw data to be downlinked first. This requires significant bandwidth, which in many cases could have been saved, for example, by pre-selecting a subset of raw data to downlink or by extracting higher-level information on-board and sending a smaller but equally relevant set of data. When it comes to housekeeping, some missions have tens of thousands of parameters and this process becomes challenging. That is why, traditionally, data processing has only been performed on-ground.Over the last decade, data analytics techniques have been developed to support operators: they automatically detect new behaviour, find recurring anomaloussituations or identify dependencies among parameters. This is time-consuming and prevents immediate reaction to identified events, whether on the payload or on theplatform itself. However, the latest generations of on-board computers open the door to extended on-board data processing. On-board data processing, e.g. usingmachine learning (ML), could allow defining autonomous actions triggered by the results of the processing.For example, ifdata is processed on-board, interesting phenomena can be detected much quicker and the spacecraft can react dynamically, for example by retargeting itself for further observations. There are many possible applications of ML and parallel computing on-board satellites, including image processing, complex onboard scheduling, autonomous decisions, etc. This will require the selection of algorithms particularly adapted to the severe resource constraints of on-boardsoftware. We propose to research both software and hardware aspects of this concept and build a solution compatible with these constraints that could beintroduced for in-flight demonstration. The proposed activity aims at investigating the possibility of using AI-based algorithms to improve the autonomy and the efficiency of the on-board management of payload data.The idea is to use raw payload data and ground-post processed data from current and past missions to realize a framework to allow the use of such data to train andtest different types of neural networks with different objectives, among which:- Generative networks for the identification of unnecessary patterns for final knowledge extractions, to allow compression of transmitted data;- Convolutional networks for segmentation/classification (e.g. clouds, shadows) segmentation adaptive to contents through to allow dynamic reduction of transmitted data;- Recurrent deep networks for the prediction and/or detection of specific events, to allow autonomous response (e.g. instrument recalibration, operative mode selection, etc.);- Sensor performance analysis for anomaly detection and timely management, and to verify the results against those obtained with current methodologies.Besides the more commonly used network topologies and associated training algorithms, consideration will be given also to the use of evolutionary programmingfor both structural and parametric definition of the networks. The development of this space-based AI application paves the way for more advanced applications, such as (e.g.) exploiting space-based video in real time for vessel tracking or sensor data fusion.The principal tasks that will be performed during the activity can be summarized as follows:- Analysis of the current state of the art regarding on-board payload data management;- Preliminary requirements definition for the framework being developed;- Identification of representative cases to be considered; Identification of available data sources and computational environments;- Implementation and use of a demonstrator of the framework;- Tests execution and preliminary results assessment;.
Directorate: Directorate of Tech, Eng. Quality
Estabilishment: ESTEC
ECOS Required: No
Classified: No
Price Range: > 500 KEURO
Contracts Officer: Anze Singer
Initiating Service: TEC-EDD
IP Measure: N/A
Prog. Reference: E/0904-611 – GSTP Element 1 Dev
Tender Type: Open Competition
Open To Tenderers From: DE+EE+GB+PL+PT
Technology Keywords: 1-A-II-Hardware Technologies for Payload Data Processing / 1-A-III-Software Technologies for Payload Data Processing
Products Keywords: 2-F-1-g-Other
If you wish to access the documents related to the Invitation to Tender, you have to log in to the ESA Portal.