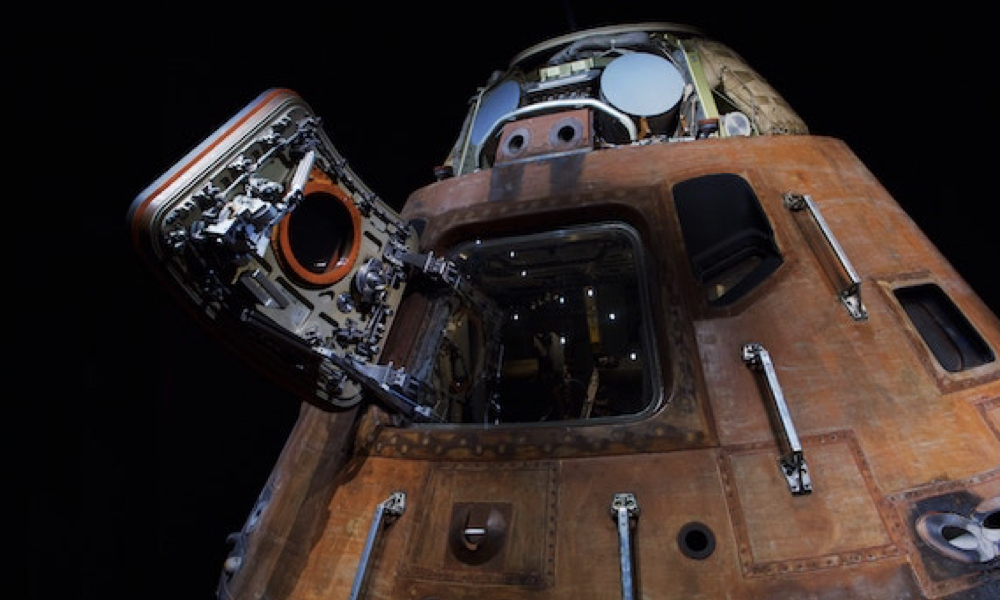
ESA Open Invitation to Tender AO10612
Open Date: 18/12/2020
Closing Date: 12/02/2021 13:00:00
Status: ISSUED
Reference Nr.: 20.1ED.12
Prog. Ref.: GSTP Element 1 Dev
Budget Ref.: E/0904-611 – GSTP Element 1 Dev
Special Prov.: BE+DE+IT+IE+RO+PL
Tender Type: C
Price Range: > 500 KEURO
Products: Satellites & Probes / On-board Data Management / On Board Data Management ¿ BB / Other
Technology Domains: Onboard Data Systems / Payload Data Processing / System Technologies for Payload Data Processing / Space System Software / Advanced Software Technologies / Advanced Software Development Methods and Tools
Establishment: ESTEC
Directorate: Directorate of Tech, Eng. & Quality
Department: Electrical Department
Division: Data Syst & Microelectronics Division
Contract Officer: Singer, Anze
Industrial Policy Measure: N/A – Not apply
Last Update Date: 18/12/2020
Update Reason: Tender issue
The objective of this activity is to design, test and prototype a generic and reusable deep learning approach for both anomaly detection isolation as well as failure prognostics.A critical function on board all spacecraft is the Failure Detection, Isolation and Recovery (FDIR) subsystem, which is vital in ensuring the safety, autonomy and availability of the system. Modern satellites complexity is increasing, and these highly complex systems require bespoke FDIR solutions with complicated architectures and difficult testability.Furthermore, traditional FDIR techniques are generally good at detecting ‘single’ failures but limited in isolation capabilities, and struggling when multiple faults combine in non-foreseen behaviours. The resulting functional unavailability and operational costs can be prohibitive for example for large fleets or constellation.The solutions developed in this activity offer the benefit of moving from a constant data downstream of all parameters (data-pull) with threshold-based alerting to a predictive alerting system that can handle seasonal effects, system noise and data correlations (information-push) scalable to very large fleets ofsatellites. These functionalities can be migrated from ground on-board the spacecraft, as the algorithms are very lean, require onlylittle computational power and are highly energy-efficient.This activity aims at demonstrating that a highly reusable, generic FDIR solution can perform satisfactorily in flight, streamlining the current FDIR development process in both time and cost whilst increasing the autonomy and availability of the next generation of spacecraft. Furthermore, it offers serious contributions towards raising the TRL of neural network implementations on space-qualified HW to flight SW standards, setting a precedent for future innovative machine learning-based applications in on-board functional avionics.By implementing embedded-AI-based analysis of on board TM time series, the failure detection results can be applied not only to trigger alarms when a fault occurs, but also for preventive maintenance. This applies both to stand alone electronics (e.g. high performance COTS FPGA, C) to generic units, assemblies, systems.The key feature of the proposed activity is the real-time condition monitoring of all spacecraft parameters. The algorithms learn from the machine itself by training on-ground during simulation/testing (e.g. hardware-in-the-loop) of the spacecraft to identify patterns in the data that characterize nominal operations. The observed patterns are used as a reference when compared to instantaneousdata. In case unusual patterns are detected, the operator is informed about identified deviations, significant statistics and correlated patterns for further analysis. A deviation manifests earlier than the fault itself. Thus, appropriate actions can be taken notonly to recover from faults, but also to prevent faults from occurring or to reduce their impact.Main tasks: – Selection of target spacecraft(s) + use case elaboration; – Development of Machine learning based FDIR algorithms with both unit/system test and qualification data and in flight data; – Verification of the algorithms performances on suitable on-board processors that will enable running deep learning applications on the edge; – Dry run on ‘ground data’ (coming from unit/system level tests) and experimentation on in-flight data on past missions; – Preparation for suitable In Orbit Demonstration for upcoming missions.
If you wish to access the documents related to the Invitation to Tender, you have to log in to the ESA Portal.